|  |
CORE 3 Driving Biological Project:
Gene Expression and the Metabolic Spectrum of Diabetes Mellitus
DBP Investigators
Principal Investigator: C. Ronald Kahn, M.D.1
Co-Investigators: Mary-Elizabeth Patti, M.D.1, Allison B. Goldfine, M.D.1, David Nathan, M.D.2
1 Research Division, Joslin Diabetes Center
2 Division of Endocrinology, Department of Medicine, Massachusetts General Hospital
1,2 Harvard Medical School, Boston, MA
Current Status: Completed 2010
1. What questions did we ask in this DBP?
We would like to know if peripheral blood gene expression profiles can be used to develop markers of disease stage and complication risk which are better than currently-available clinical markers of glucose control. Moreover, we would like to develop precise, noninvasive markers of insulin resistance and type 2 diabetes risk which mirror data from research-based, invasive muscle biopsy samples – so that aggressive preventive strategies, both lifestyle and pharmacologic, can be directed at high-risk individuals.
2. How did i2b2 help us answer these questions?
The collaborative opportunities between informatics/computational biology experts and leading academic clinicians within i2b2 are critical for efficiently developing novel, non-invasive, scientifically based strategies for diabetes risk assessment and, ultimately, targeted prevention and treatment strategies.
3. What tools were developed from our work that will be of value to others?
We anticipate that data sets derived from the Diabetes Driving Biology Project, publicly released on the website, will be used as a standard to identify genes uniquely related to glucose control and/or risk for diabetes complications. We are hopeful that these data will also eventually lead to generation of assays and models to improve risk assessment, and targeted treatment.
4. What new clinical discoveries arose from our i2b2?
We ultimately hope that data analysis of precisely obtained clinical samples, in the context of interactions between clinician-investigators and computational biologists, will lead to the identification of not only improved risk assessment for prediabetes and diabetes, but also identification of novel genes and proteins important in the pathogenesis of type 2 diabetes and/or diabetes complications.
Background and Specific Aims:
Both genetic and environmental factors play important roles in the pathogenesis of diabetes. In type 1 diabetes, autoimmune destruction of pancreatic b-cells results in insulin deficiency, hyperglycemia, and ketoacidosis. Type 2 diabetes is characterized by both insulin resistance and relative insulin deficiency. In both forms of diabetes, there are multiple other abnormalities of lipid and protein metabolism, and as a result of the combined effects of these abnormalities, patients are at risk for long-term complications of disease. Microvascular complications include diabetic retinopathy, nephropathy and neuropathy; in larger blood vessels, diabetes may lead to premature atherosclerosis, myocardial infarction, stroke, and amputation.
Recent studies in patients with both type 1 and 2 diabetes show that improved control of hyperglycemia and associated metabolic abnormalities reduces risk of long-term complications. While it is now possible to measure blood glucose frequently and to estimate chronic glucose control, the precise effects of both insulin resistance and hyperglycemia on the metabolic and genetic processes which precede diabetes complications remain impossible to measure clinically. In addition, in type 2 diabetes, insulin resistance and its associated cardiovascular risk (“metabolic syndrome”) precede the diagnosis of diabetes by many years, making early metabolic staging, diagnosis and intervention a public health imperative. Thus, the ability to accurately characterize gene expression profiles, and to integrate these data with clinical physiological parameters, now offers an opportunity to measure the effects of altered metabolic control, as affected by disease predisposition or medical therapy, at the cellular and nuclear level.
Our hypothesis is that gene expression analysis will permit development of markers of disease stage and complication risk which are better correlated with clinical outcomes and metabolic status than direct and indirect measures of glucose itself. The overall goal of this project is to identify alterations in expression in clinically accessible samples in type 1 and type 2 diabetes, and to determine to what extent this parallels the metabolic derangements of diabetes and the development of diabetes-specific complications (Figure 1).
The specific aims are:
1. To compare expression in mononuclear cells, a nonclassical insulin target tissue which can be obtained noninvasively, with that of muscle, a primary insulin target tissue not accessible clinically, in humans with and without insulin resistance and diabetes. With these data, we will define gene sets expressed in mononuclear cells which can serve as a proxy for muscle gene expression and a clinically useful marker for both insulin resistance and diabetes.
2. To evaluate the impact of enhanced metabolic control on gene expression profiles in circulating mononuclear cells from patients with type 1 or type 2 diabetes.
3. To determine if gene expression in circulating mononuclear cells can define the long-term effects of metabolic control (“metabolic memory”) and risk for diabetes-related complications in previous tightly-controlled and poorly-controlled diabetic patients in the DCCT/EDIC population.
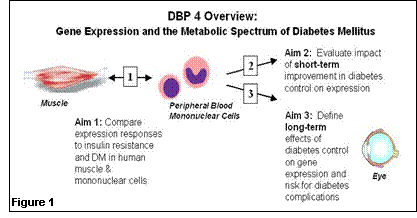
Update:
· We completed recruitment, metabolic characterization, and muscle biopsy and white blood cell collection of a study cohort which included subjects in 3 experimental groups: 18 insulin sensitive, 23 insulin resistant (normoglycemic and ~ “prediabetic”), and 11 with type 2 diabetes (elevated fasting glucose and hemoglobin A1c). For all subjects, RNA was harvested from muscle and white blood cells in the fasting state; cRNA was prepared and hybridized to Affymetrix U133 2.0 human arrays.
· Within the current cohort, GSEA analysis demonstrated modest reduction in expression of OXPHOS genes in individuals with type 2 diabetes (p<0.01, FDR 0.13) – but NOT in insulin resistant subjects Since understanding whether impaired mitochondrial function contributes to diabetes risk is a key question in pathogenesis and development of new therapeutics, we have addressed this issue within this dataset using several informatics approaches:
(1) Unsupervised clustering of gene expression for OXPHOS genes,
(2) Principal components analysis,
(3) Gene network enrichment analysis (GNEA),
(4) Correlation analysis of gene expression:
Our findings indicate that OXPHOS is a highly coregulated set of genes, for which expression is modestly reduced in overt diabetes, but not in insulin resistant or “prediabetes” states. These data would suggest that OXPHOS gene expression per se is unlikely to play a major pathogenic role in insulin resistance induction, and instead may be secondary to the diabetes metabolic milieu in muscle.
Taken together, these data strongly suggest that alternative mechanisms may contribute to patterns of gene expression in humans with insulin resistance and diabetes risk. Quantitative RT-PCR analysis to confirm our other top-ranking findings at an individual gene level is in progress.
· We have begun cross-tissue comparison of expression patterns using both (1) visual identification of concordant gene expression patterns using heat maps of gene set enrichment scores, and (2) identification of gene sets correlated between tissues which also correlate with metabolic variables. While our sample number for peripheral lymphocytes (15) is lower than that of muscle, it is intriguing that the gene sets identified as highly ranked with insulin sensitivity in muscle were also positively correlated with SI in mononuclear cells. We are in the process of selecting representative genes for PCR validation of these interesting patterns.
· To evaluate the impact of short-term (8 weeks) improvement in metabolic control on gene expression profiles in circulating white blood cells from patients with uncontrolled type 1 diabetes, we recruited subjects with type 1 diabetes into 2 study groups: (1) poor glycemic control at entry (2) good glycemic control at entry At the end of the study period, subjects in the intervention group improved glycemia remarkably, to 7.7%, while those in the no-intervention group remained stable at 7.0%. Gene set enrichment analysis demosntrated, to our surprise, that OXPHOS gene expression was increased in poorly controlled type 1 diabetes (nominal p <0.001, FWER 0.01). Also interesting was the finidng that PBX1 correlated well with glycemic control at baseline. PCR analysis is underway.
Publications:
- Liu M, Liberzon A, Kong SW, Weil RL, Park PJ, Kohane IS, Kasif S. Network-based analysis of affected biological processes in Type 2 diabetes models. PLoS Genetics. 2007;3:0001-0015. doi:10.1371/journal.pgen.0030096.
- Doria A, Patti ME, Kahn CR. The emerging genetic architecture of type 2 diabetes. Cell Metabolism 2008;8(3); 186-200.
- Isganaitis E, Jimenez-Chillaron J, Woo M, Chow A, DeCoste J, Vokes M, Liu M, Kasif S, Zavacki AM, Leshan RL, Myers MG, Patti ME. Accelerated postnatal growth increases lipogenic gene expression and adipocyte size in low-birth weight mice. Diabetes. 2009;May;58(5):1192-200. PMID: 19208909
- Pihlajamaki J, Boes T, Kim EY, Dearie F, Kim BW, Schroeder J, Mun E, Nasser I, Park PJ, Bianco AC, Goldfine AB, Patti ME. Thyroid hormone-related regulation of gene expression in human fatty liver. J Clin Endo Metab. 2009;94:3521-8.
- Jin W, Patti ME. Genetic determinants and molecular pathways in the pathogenesis of diabetes. Clinical Science. 2009;116 (2): 99-111. PMID:19076063.
- Patti ME , Corvera S. The role of mitochondria in the pathogenesis of Type 2 Diabetes. Endocrine Reviews. 2010;31(3)364-95. PMID:20156986.
- Pihlajamaki J, Itkonen P, Crunkhorn S, Vänttinen M, Dearie F, Boes T, Jimenez-Chillaron J, Lappalainen T, Miettinen P, Park P, Nasser I, Goldfine AB, Laakso M, Patti ME. Expression of splicing factor genes is reduced in human obesity: links to altered Lipin 1 splicing and enhanced lipogenesis. Cell Metabolism. 2011;14(2):208-18. PMID:21803291.
|  |